Deep Learning :
Published on October 21, 2022
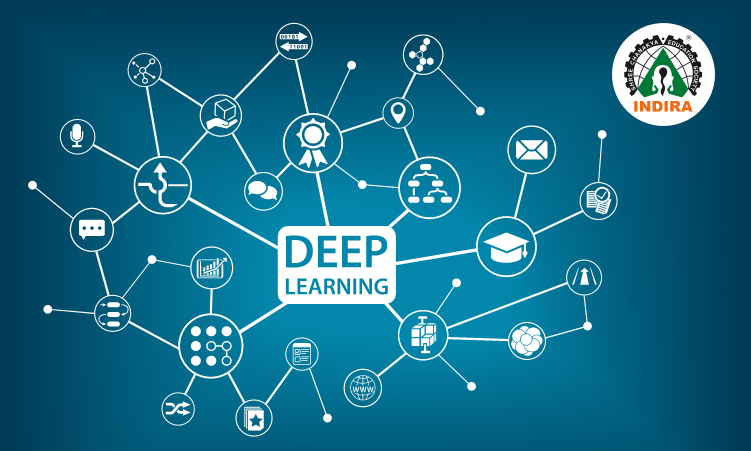
People learn from experiences. The richer our experiences, the more accurate we can take decisions. In the Artificial Intelligence (AI) discipline known as deep learning, the experiences through which machines can learn are defined by the data they acquire, and the quantity and quality of data determine how much they can take decisions.
Deep learning is a branch of machine learning. Many traditional machine learning algorithms, which have a finite capacity to learn no matter how much data they acquire, deep learning systems can improve their performance with access to more data: the machine version of more experience. Deep Learning has many applications such as driving a car, detecting weeds in a field of crops, detecting diseases, and so on.
For many tasks, such as computer vision, natural language processing (NLP), machine translation, and robotics, the performance of deep learning systems is much higher than that of conventional machine learning systems.
Deep Learning use case examples
Robotics
Many of the recent developments in robotics have been driven by advances in AI and deep learning. AI enables robots to sense and respond to their environment. This capability increases the range of functions they can perform, from navigating their way around warehouse floors to sorting and handling objects that are uneven, fragile, or jumbled together. Something as simple as picking up a strawberry is an easy task for humans, but it has been remarkably difficult for robots to perform. As AI progresses, that progress will enhance the capabilities of robots.
Agriculture
AI gives more potential to farming. Today, deep learning enables farmers to deploy equipment that can see and differentiate between crop plants and weeds. This capability allows weeding machines to selectively spray herbicides on weeds and leave other plants untouched. Farming machines that use deep learning–enabled computer vision can even optimize individual plants in a field by selectively spraying herbicides, fertilizers, fungicides, insecticides, and biologicals. In addition to reducing herbicide use and improving farm output, deep learning can be further extended to other farming operations such as applying fertilizer, performing irrigation, and harvesting.
Medical imaging and healthcare
Deep learning has been particularly effective in medical imaging, due to the availability of high-quality data and the ability of convolutional neural networks to classify images. For example, deep learning can be as effective as a dermatologist in classifying skin cancers, if not more so. Several vendors have already received FDA approval for deep learning algorithms for diagnostic purposes, including image analysis for oncology and retina diseases. Deep learning is also making significant inroads into improving healthcare quality by predicting medical events from electronic health record data.
Blog by :
Dr. Vikas Nandgaonkar,
ICEM, Pune.
Mechanical engineering, Indira COE.
Posted in:Computer Engineering, Education
EDUCATION IS REAL GOLD

It is health that is real wealth and not pieces of gold and silver.
September 23, 2021
Criteria for Engineering Admission in 2020
May 9, 2020
Everything You Need To Know: MCA after BCom!
July 21, 2020
Education is real Gold
October 16, 2021